카테고리 없음
python(61)- Segmentation(객체 분할)
두설날
2024. 8. 13. 17:40
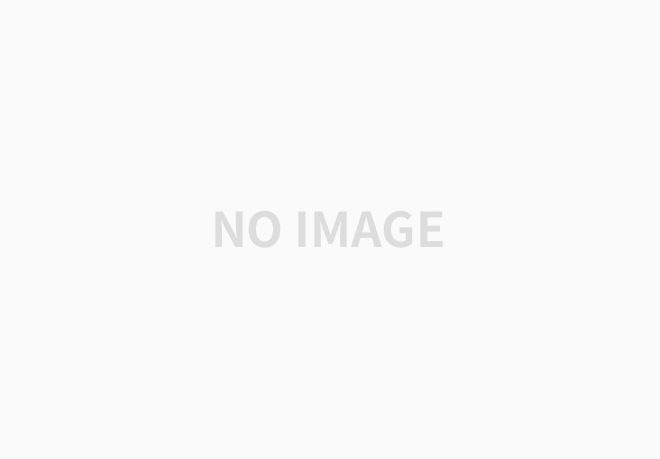
*이 글을 읽기전에 작성자 개인의견이 있으니, 다른 블로그와 교차로 읽는것을 권장합니다.*
1. Image Segmentation
- 컴퓨터 비전 분야에서 이미지나 비디오의 디지털 데이터를 여러 개의 부분 또는 객체로 분할하는 기술
- 이미지의 중요한 요소들을 식별하고 각 요소를 개별적으로 분석할 수 있게 하는 것
2. Image Segmentation의 유형
- Semantic Segmentation
- 이미지의 각 픽셀을 미리 정의된 클래스 레이블 중 하나로 분류
- 예) 자율 주행차의 도로, 차선, 보행자 등을 식별
- Instance Segmentation
- 동일한 클래스 내의 서로 다른 개체들을 개별적으로 식별
- 예) 이미지 내의 있는 개별 물체의 수를 파악하고 각각 물체를 식별 및 추적하는 경우
- Panoptic Segmentation
- Semantic Segmentation, Instance Segmentation을 결합한 형태
- 배경과 같은 클래스를 처리하는 Semantic Segmentation과 개체를 구분하는 Instance Segmentation을 모두 수행
- 예) 풍경 이미지에서 하늘, 도로, 나무와 사람, 자동차를 동시에 식별
!pip install ultralytics
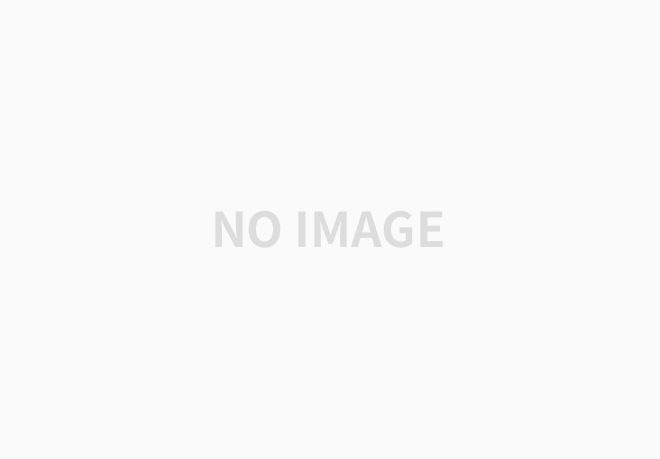
import os
import random
import shutil
import cv2
import glob
import json
import numpy as np
import matplotlib.pyplot as plt
from tqdm import tqdm
다음과 같은 데이터셋 경로 설정
data_root
data_root/data
data_root/cd/train -> images, labels
data_root/cd/valid -> images, labels
data_root/cd/test -> images, labels
# 데이터 불러오기
data_root = '/content/drive/MyDrive/KDT/8. 컴퓨00000000000000터 비전/9. Segmentation'
file_root = f'{data_root}/data'
cls_list = ['Scratched', 'Breakage', 'Separated', 'Crushed']
project_name = 'cd'
train_root = f'{data_root}/{project_name}/train'
valid_root = f'{data_root}/{project_name}/valid'
test_root = f'{data_root}/{project_name}/test'
for folder in [train_root, valid_root, test_root]:
if not os.path.exists(folder):
os.mkdir(folder)
for s in ['images', 'labels']:
s_folder = f'{folder}/{s}'
if not os.path.exists(s_folder):
os.mkdir(s_folder)
# 라벨링 형태 변환(YOLO): [cls xc, yc, w, h]
# [[x1, y1], [x2, y2] ... ] -> [cls xc yc w h] : normalize
def json_to_yolo_polygon(polygon, w, h):
yolo_list = []
for p in polygon:
yolo_list.append(p[0]/w)
yolo_list.append(p[1]/h)
return " ".join([str(x) for x in yolo_list])
file_list = glob.glob(f'{file_root}/annotations/*.json')
random.seed(2024)
random.shuffle(file_list)
print(len(file_list))
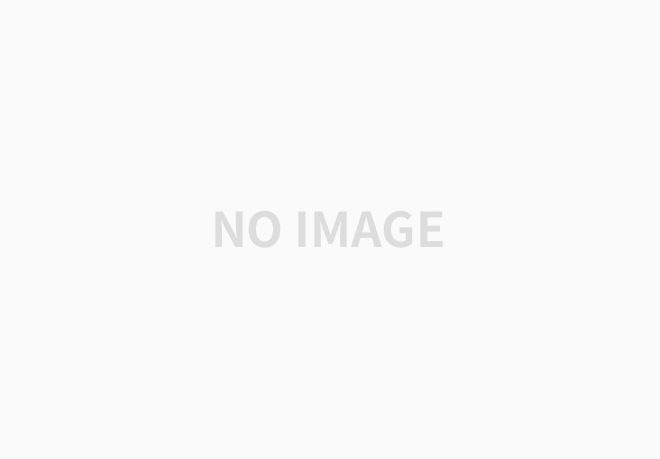
# 라벨링 형태 변환 파일 생성(json -> txt)
if not os.path.isdir(f'{file_root}/labels'):
os.mkdir(f'{file_root}/labels')
for file in tqdm(file_list):
result = []
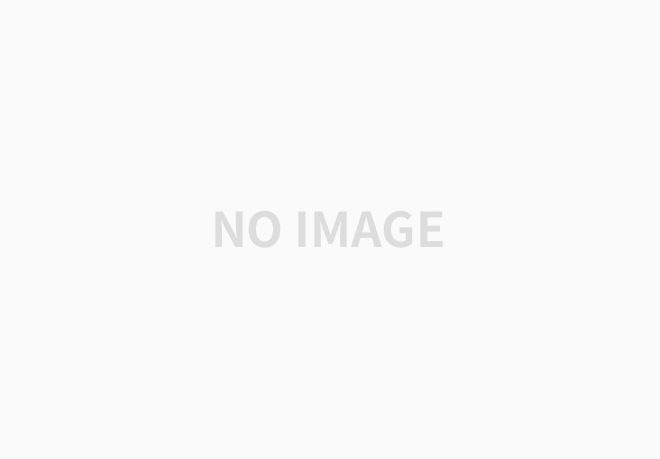
# 라벨링 형태 변환 파일 생성(json -> txt)
if not os.path.isdir(f'{file_root}/labels'):
os.mkdir(f'{file_root}/labels')
for file in tqdm(file_list):
result = []
with open(file, 'r') as json_file:
data = json.load(json_file)
h = data['images']['height']
w = data['images']['width']
for ann in data['annotations']:
label = ann['damage']
if label in cls_list:
polygon_cood = ann['segmentation'][0][0][:-1]
cood_string = json_to_yolo_polygon(polygon_cood, w, h)
yolo_string = f'{cls_list.index(label)} {cood_string}'
result.append(yolo_string)
if result:
save_path = file.replace('annotations', 'labels').replace('json', 'txt')
with open(save_path, 'w', encoding='utf-8') as f:
f.write('\n'.join(result))
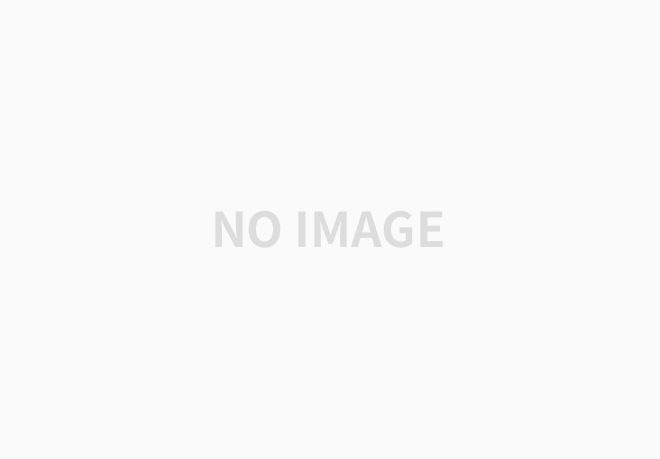
file_list = glob.glob(f'{file_root}/labels/*.txt')
random.shuffle(file_list)
test_ratio = 0.1
num_file = len(file_list)
test_list = file_list[:int(num_file*test_ratio)]
valid_list = file_list[int(num_file*test_ratio):int(num_file*test_ratio)*2]
train_list = file_list[int(num_file*test_ratio)*2:]
for i in test_list:
label_name = i.split('/')[-1]
shutil.copyfile(i, f'{test_root}/labels/{label_name}')
img_name = i.split('/')[-1].replace('txt', 'jpg')
img_path = f'{file_root}/images/{img_name}'
shutil.copyfile(img_path, f'{test_root}/images/{img_name}')
for i in valid_list:
label_name = i.split('/')[-1]
shutil.copyfile(i, f'{valid_root}/labels/{label_name}')
img_name = i.split('/')[-1].replace('txt', 'jpg')
img_path = f'{file_root}/images/{img_name}'
shutil.copyfile(img_path, f'{valid_root}/images/{img_name}')
for i in train_list:
label_name = i.split('/')[-1]
shutil.copyfile(i, f'{train_root}/labels/{label_name}')
img_name = i.split('/')[-1].replace('txt', 'jpg')
img_path = f'{file_root}/images/{img_name}'
shutil.copyfile(img_path, f'{train_root}/images/{img_name}')
project_root = '/content/drive/MyDrive/KDT/8. 컴퓨터 비전/9. Segmentation'
%cd /content/drive/MyDrive/KDT/8. 컴퓨터 비전/9. Segmentation
import yaml
import ultralytics
from ultralytics import YOLO
ultralytics.checks()
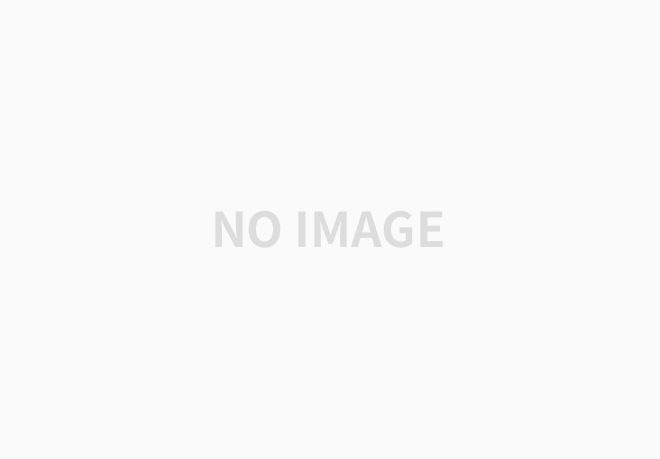
data = dict()
data['train']= train_root
data['val'] = valid_root
data['test'] = test_root
data['nc'] = len(cls_list)
data['names'] = cls_list
with open (f'{project_root}/car_damage.yaml', 'w') as f:
yaml.dump(data, f)
model = YOLO('yolov8n-seg.yaml')
results = model.train(data = 'car_damage.yaml', epochs = 100, batch=16, device=0, patience=30, name='yolo_s')
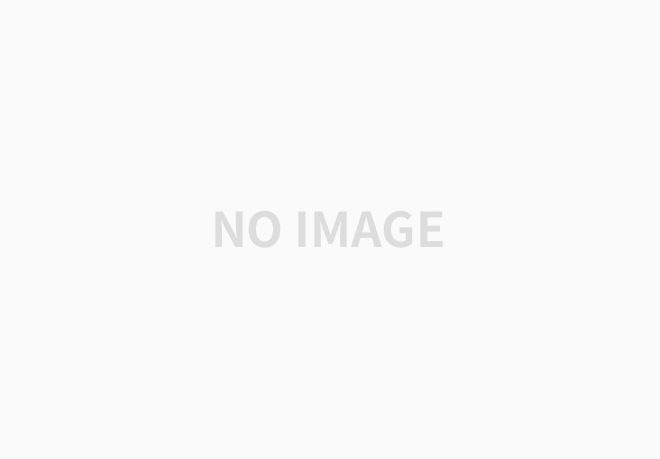
%cd /content/drive/MyDrive/KDT/8. 컴퓨터 비전/9. Segmentation
project_root = '/content/drive/MyDrive/KDT/8. 컴퓨터 비전/9. Segmentation'
result_folder = f'{project_root}/runs/segment'
model = YOLO(f'{result_folder}/yolo_s/weights/best.pt')
metrics = model.val(split='test')

%cd /content/drive/MyDrive/KDT/8. 컴퓨터 비전/9. Segmentation
test_file_list = glob.glob(f'{test_root}/images/*')
random.shuffle(test_file_list)
test_img = cv2.imread(test_file_list[0])
img_src = cv2.cvtColor(test_img, cv2.COLOR_BGR2RGB)
result = model(img_src)[0]

from skimage.draw import polygon2mask
test_img = cv2.imread(test_file_list[11])
img_src = cv2.cvtColor(test_img, cv2.COLOR_BGR2RGB)
result = model(img_src)[0]
result_mask = np.zeros(test_img.shape[:2])
masks = result.masks
for m in masks:
polygon_coord = m.xy[0]
# 주어진 이미지 크기와 폴리곤 좌표를 사용하여 해당 영역을 1로 채운 마스크를 생성
# 나머지는 0으로 유지
mask = polygon2mask(test_img.shape[:2], polygon_coord)
# maximum(): 두 배열의 요소별 최대값을 반환하므로 여러 객체의 마스크가 겹치더라도 최대값을 유지
result_mask = np.maximum(mask, result_mask)
# 2D 마스크를 3D 배열로 변환하고 repeat() 사용해 동일한 값을 3개의 채널에 복사
result_mask = np.repeat(result_mask[:, :, np.newaxis], 3, -1)
plt.subplot(1, 2, 1)
plt.imshow(img_src)
plt.subplot(1, 2, 2)
plt.imshow(result_mask)
plt.show()

%cd /content/drive/MyDrive/KDT/8. 컴퓨터 비전/9. Segmentation
project_root = '/content/drive/MyDrive/KDT 시즌3/8. 컴퓨터 비전/9. Segmentation'
result_folder = f'{project_root}/runs/segment'
test_img = cv2.imread(test_file_list[13])
img_src = cv2.cvtColor(test_img, cv2.COLOR_BGR2RGB)
result = model(test_img)[0]
result_mask = np.zeros(test_img.shape[:2])
masks = result.masks
for m in masks:
polygon_coord = m.xy[0]
# 주어진 이미지 크기와 폴리곤 좌표를 사용하여 해당 영역을 1로 채운 마스크를 생성
# 나머지는 0으로 유지
polygon_coord = np.array([[p[1], p[0]] for p in polygon_coord])
mask = polygon2mask(test_img.shape[:2], polygon_coord)
# maximum(): 두 배열의 요소별 최대값을 반환하므로 여러 객체의 마스크가 겹치더라도 최대값을 유지
result_mask = np.maximum(mask, result_mask)
# 2D 마스크를 3D 배열로 변환하고 repeat() 사용해 동일한 값을 3개의 채널에 복사
result_mask = np.repeat(result_mask[:, :, np.newaxis], 3, -1)
plt.subplot(1, 2, 1)
plt.imshow(img_src)
plt.subplot(1, 2, 2)
plt.imshow(result_mask)
plt.show()

polygon_coord